From the moment the text analysis assignment was mentioned, I knew I wanted to do something with transcripts from the TV series Buffy the Vampire Slayer (seven seasons airing from March 10, 1997, to May 20, 2003). I had no idea what I wanted to examine or any question I might want to answer, but it’s a show I love and have seen many times, and I figured it would just be fun.
Text Gathering
I decided to do a “bag of words” comparison in Voyant using the Cirrus word cloud tool, and I originally expected to use each of the seven season finale episodes as my texts, assuming that a season finale encapsulates the overall themes from the entire season and I might be able to identify some kind of series-wide arch. But some finales were two-parters, so the amount of text being compared across seasons wouldn’t be the same, which I thought could be a problem. Inspired by our class conversation last week as we tried to answer the question “What is text?,” I realized there are several episodes of BTVS that play with some of the concepts we were discussing. So I decided to play around with the transcripts from select episodes instead to see what I might learn. I had wanted to take all of my transcripts from the same source, but this proved problematic as not every archive had complete transcripts for every episode. For the first two episodes I chose, I got the transcribed text from angelfire.com. For the latter two episodes I chose, I got the transcribed text from transcripts.foreverdreaming.org. None of these are official transcripts.
I initially copied the text for each episode into a word document, as I wanted to make sure there wasn’t any hidden metadata creeping into the text that might distort my visualizations/analyses. Some of the transcripts including stuff like “ACT I” or “Commercial Break,” both of which I removed. I was initially worried about the scene/stage directions being highly subjective as they were written by different fans (and not from the original scripts used in production) and also about the “Name: dialogue” format for the lines. But I figured most of this type of text also exists in other narratives like books when the author is setting scenes and defining who is speaking. However, when I put in the text for each episode, all of my word clouds were primarily just the names of the main characters and the most prominent secondary characters in each episode, which didn’t really seem very interesting in terms of analysis potential. So I then went back into each of the transcripts and removed the names of the four main characters (Buff, Willow, Xander, and Giles) as well as any of the other characters who are prominent in the series or were just prominent in that episode (e.g., Ms. Calendar, Angel, Spike, Anya, Oz, Tara, Dawn, Riley, Wesley, Jonathan, etc.). I also expanded the word clouds to include the top 155 words from each episode.
I Robot, You Jane
The first episode I chose is “I Robot, You Jane” (season 1, episode 8) in which a demon, Moloch the Corrupter, that had been imprisoned inside a book is released into the internet when the book is scanned as part of a digital archiving initiative at the school. Rupert Giles, the school librarian, gets into an argument with the computer science teacher Ms. Calendar, who is leading the initiative, at the beginning of the project:
Ms. Calendar: Oh, I know, our ways are strange to you, but soon you will join us in the 20th century. With three whole years to spare! (grins)
Giles: (smugly) Ms. Calendar, I’m sure your computer science class is fascinating, but I happen to believe that one can survive in modern society without being a slave to the, um, idiot box.
Ms. Calendar: (annoyed) That’s TV. The idiot box is TV. This (indicates a computer) is the good box!
Giles: I still prefer a good book.
Fritz: (self-righteously) The printed page is obsolete. (stands up) Information isn’t bound up anymore. It’s an entity. The only reality is virtual. If you’re not jacked in, you’re not alive. (grabs his books and leaves)
Ms. Calendar: Thank you, Fritz, for making us all sound like crazy people. (to Giles) Fritz, Fritz comes on a little strong, but he does have a point. You know, for the last two years more e-mail was sent than regular mail.
Giles: Oh…
Ms. Calendar: More digitized information went across phone lines than conversation.
Giles: That is a fact that I regard with genuine horror.
http://www.angelfire.com/ny4/amai/Buffy/s1ep8.html
Several scenes later, their argument continues:
Ms. Calendar: (exasperated) You’re a snob!
Giles: (incredulous) I am no such thing.
Ms. Calendar: Oh, you are a big snob. You, you think that knowledge should be kept in these carefully guarded repositories where only a handful of white guys can get at it.
Giles: Nonsense! I simply don’t adhere to a, a knee-jerk assumption that because something is new, it’s better.
Ms. Calendar: This isn’t a fad, Rupert! We are creating a new society here.
Giles: A society in which human interaction is all but obsolete? In which people can be completely manipulated by technology, well, well… Thank you, I’ll pass.
http://www.angelfire.com/ny4/amai/Buffy/s1ep8.html
The episode aired in 1997, and Giles’s character is generally portrayed as a technophobe throughout the series. His latter argument against technological innovation as good only because of its newness and against technology being necessarily the direction of “progress” reminds me of Johanna Drucker’s “Pixel Dust: Illusions of Innovation in Scholarly Publishing.” And Ms. Calendar is clearly trying to promote technology and digital archives as means to open access and inclusion. Does any of this come through in the word cloud? I think a little bit, with computer and book coming through as two of the highest used words (at 39 and 37, respectively). Cut is the most used word at 93, but I’m fairly sure that is because of stage directions.
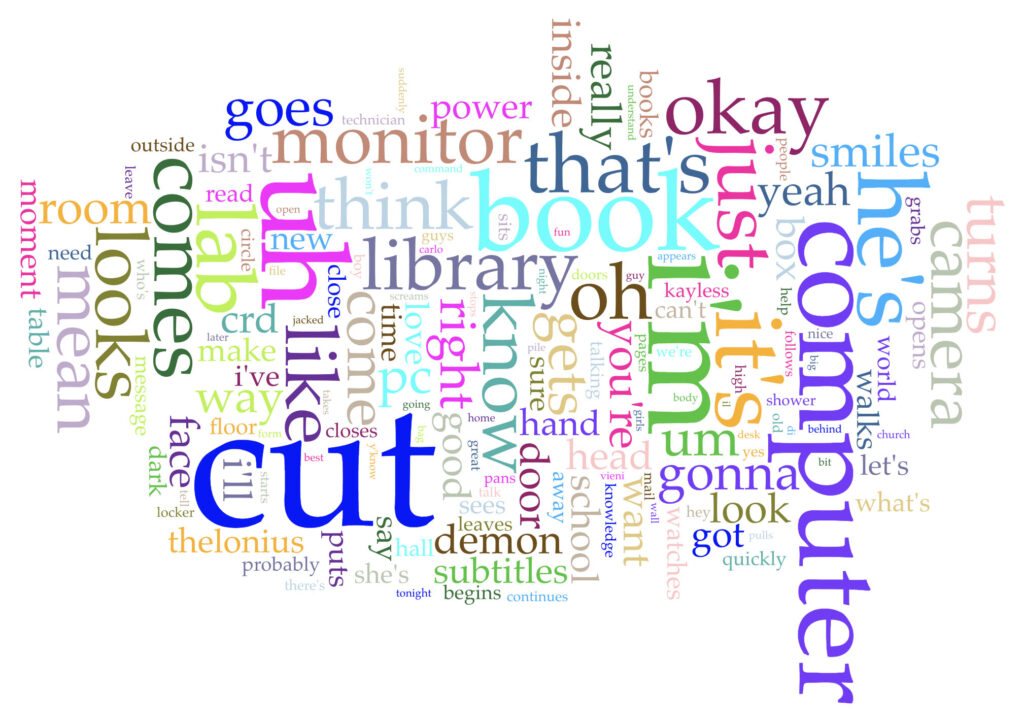
Earshot
The second episode I chose is “Earshot” (season 3, episode 18). In this episode Buffy is fighting some demons, per usual, but something goes wrong when some bodily fluid from a demon is absorbed through her hand. She is infected with “an aspect of the demon,” which turns out to be an ability to hear other people’s thoughts. At first this is exciting as Buffy hears what other people are thinking of her and finds out interesting secrets people are keeping; however, the power grows and grows to the point at which it is driving her mad because she is hearing everything from everyone and cannot distinguish any of it. I thought of this episode when we were debating in class whether everything could be text and an example given was everything that people say, whether or not it is recorded or preserved. As we touched on text being understood through symbols, I thought words that we see only in our minds but do not share, either written or aloud, could also be text. This episode also felt like an apt analogy to what it might feel like for a human mind to analyze text on the same scale as a computer. What does this word cloud show? Looks, look, and looking are all very prominent, as are thoughts and demon. Cut again is very high, but this again I think is attributable to the stage directions.
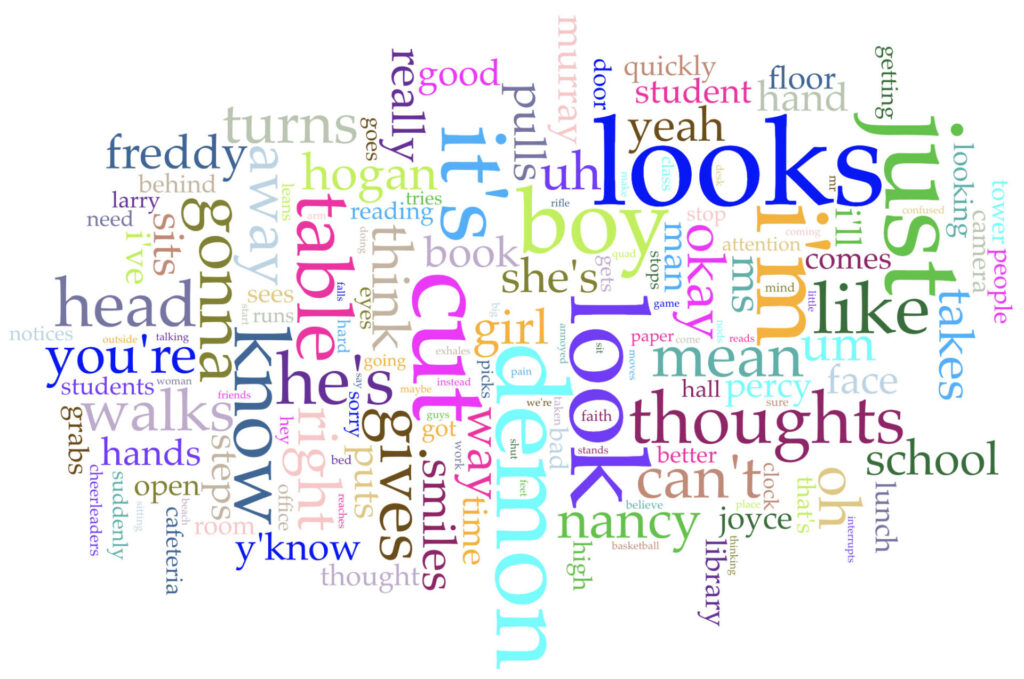
Hush
The third episode I chose is “Hush” (season 4, episode 10). In this episode, the town of Sunnydale is the setting for a gristly fairy tale, where the Gentlemen come in and silence everyone so that nobody can scream as they harvest the requisite number of hearts to terrorize humanity another day. So here we have an episode (and text) that is essentially quiet, with communication limited to succinct phrases and crude pictures easily written/drawn on portable white boards and the nonverbal—body language and pantomime. Are these forms of communication text? I’m inclined to say yes as ultimately an entire storyline is conveyed to the audience just as it is for any other episode of the show. Given that this episode has the least amount of dialogue and relies the most on stage directions, which were written by a fan and are not from the original script, I’m guessing this word cloud says more about the word preferences of the specific person who wrote this transcript more so than any of the other word clouds (though likely the original script would do the same of those writers, and now I’m wondering whether my assumption of a single author is even remotely accurate as each episode in the show and across the series would have had multiple and at times different authors). Gentlemen/Gentleman are both relatively dominant in this word cloud, as well as lackey/lackeys (who assist the Gentlemen). Looks is also high up there again, and picture and talk seem to be about the same size, though I think talk is present here because of its absence, especially as can’t is also very prominent (“Can’t even talk/can’t even cry” is part of the Gentlemen’s grim fairy tale rhyme).
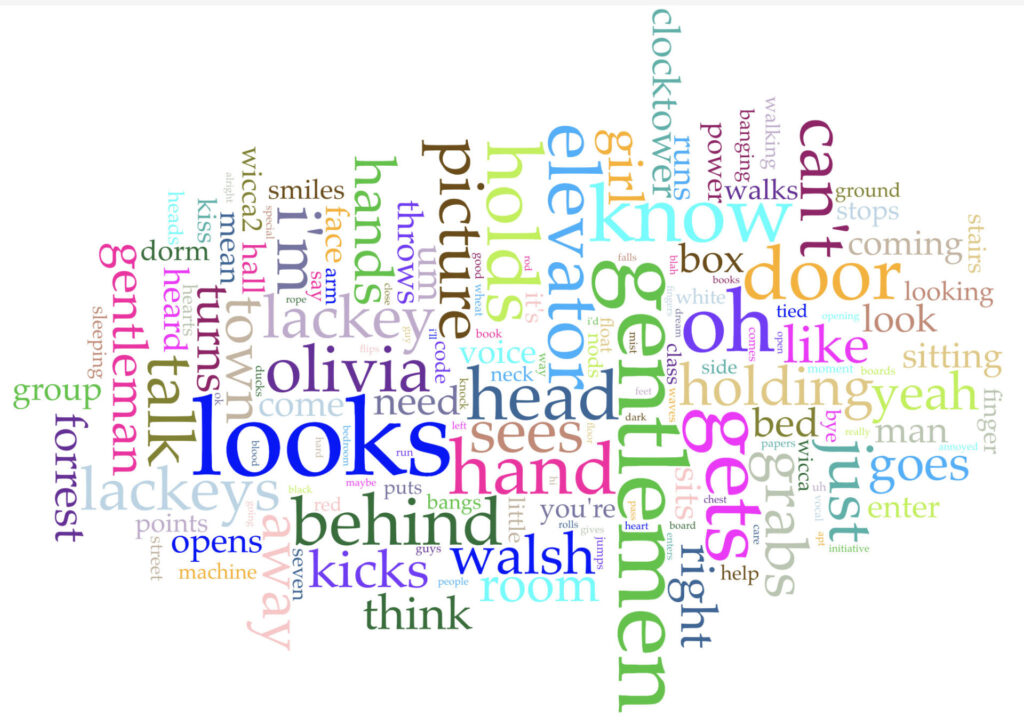
Once More, with Feeling
The last episode I chose is “Once More, with Feeling” (season 6, episode 7). The musical episode! This time all of Sunnydale is under the spell of a demon who forces everyone to communicate through song and dance. It’s a feast for the eyes and the ears. Someone in class had discussed sheet music as text, and certainly song lyrics are text. (Would choreography also count as text?) Going off of that, then, this episode would contain two texts (three?) actually, the music as well as the lyrics. How do these texts “speak” with and inform one another? How is the message conveyed differently? I’m not sure this word cloud can really measure that as it only represents the lyrical text and not the musical text. Sweet is by far the most used word (65); looks and looking feature prominently again (are these stage directions or spoken?). Song, singing, and dancing are also high up there, and I can see other music-related terms like musical, refrain, sing, sings, dances, music, rock. I’m happy to see that bunnies also makes the cut for this cloud (because “It must be BUNNIES!”).
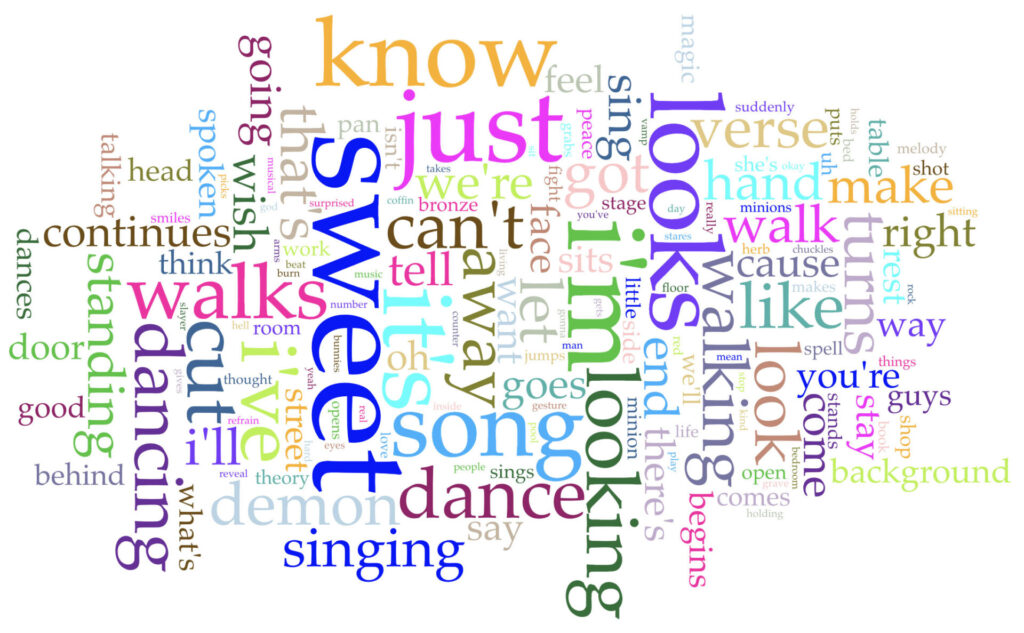
Future Text Mining
What did I learn here? I’m not entirely sure. Also, I had all of my Voyant tabs open for a while, and I noticed that the layout of the word clouds kept shifting (like the stairs at Hogwarts). In checking my word cloud links, the displays have even changed from the versions I took sceenshots of (WHY?). This makes me even more confused about how Voyant determines the layout of this particular visualization tool and how useful it is to compare different words clouds against each other. Going forward I’d be curious to know how these clouds might look differently if I were working from the finalized scripts used for producing each episode. I’m also curious to dig a little deeper into the difference between stage directions and spoken dialogue. Do we need to distinguish them? It seems like they both ultimately work together to tell the story of each episode. Are there visual cues in the final episodes that are not represented in the stage directions? What does that say about translating text into images and vice versa?